DrugTar Algorithm
We developed "DrugTar", a novel deep learning framework that integrates pre-trained sequence embeddings and GO term for predicting druggability. The overview of this method is shown here:
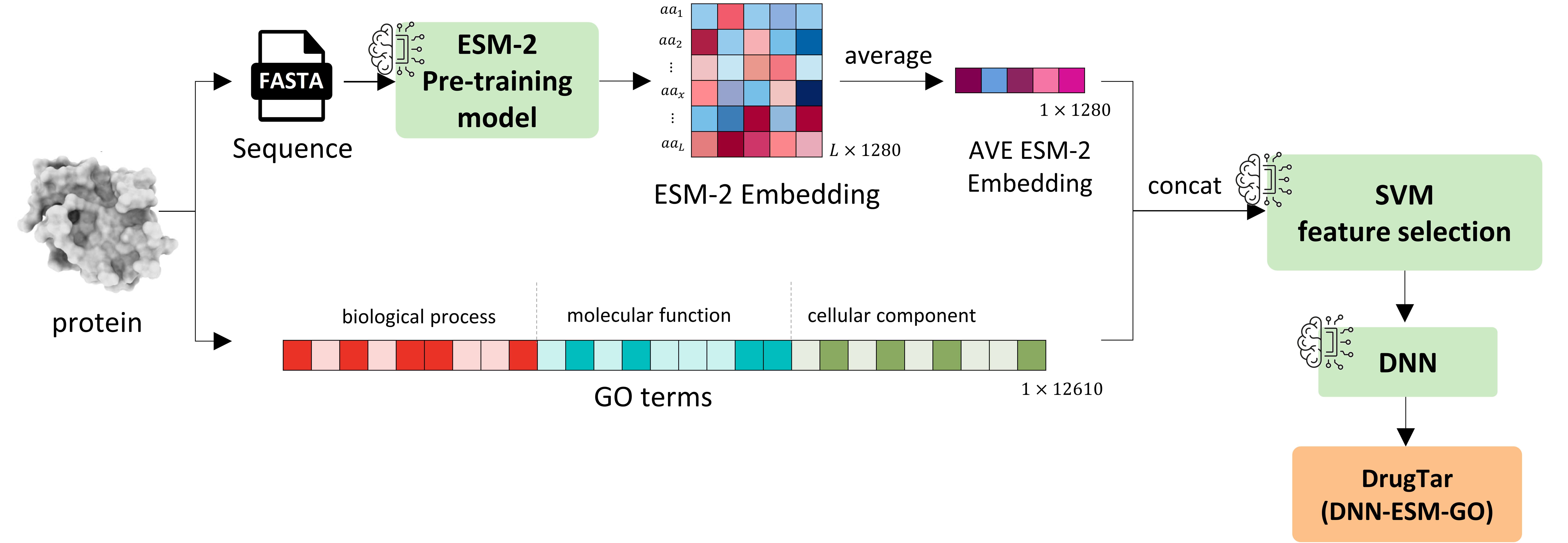
DrugTar leverages the combined power of the ESM-2 embedding method and GO annotations within a deep neural network framework. Effective feature selection in biological systems with high-dimensional features is critical to improving classification accuracy and preventing overfitting. Therefore, the SVM feature selection algorithm was employed on the concatenation of ESM-2 embedding and GO terms in DrugTar. Subsequently, a deep neural network with three hidden layers was utilized to predict protein druggability. DrugTar achieved areas under the curve and precision-recall curve values above 0.90, outperforming state-of-the-art methods. In conclusion, DrugTar streamlines target discovery as a bottleneck in developing novel therapeutics. For more information, see DrugTar Improves Druggability Prediction by Integrating Large Language Models and Gene Ontologies.
How to Cite?
If you find this tool useful in your research, please cite this paper:
DrugTar Improves Druggability Prediction by Integrating Large Language Models and Gene Ontologies